

Most significant is the potential deployment of real-time interactive experiences that are designed to persuade, coerce, or manipulate users as a form of AI-powered targeted influence. While this will unleash many positive applications, there is significant danger of abuse. When combined, these two disciplines will enable users to hold conversations with realistic virtual agents. Commonly referred to as “Conversational AI,” this technology has advanced rapidly with the deployment of Large Language Models (LLMs). The first area of advancement has been virtual and augmented worlds, now commonly called “The Metaverse.” The second area of advancement has been the foundational AI models that allow users to freely interact with computers through natural dialog. Over the last 18 months, two human-computer interaction (HCI) technologies have rapidly come to mainstream markets, funded by massive investments from major corporations. We conclude by reflecting on implications of our work for anticipating algorithmic harms from emerging systems. Third, we discuss important harms from increasingly agentic systems and ways forward for addressing them. Second, we argue for the need to anticipate harms from increasingly agentic systems. First, we explain the notion of an increase in agency for algorithmic systems in the context of diverse perspectives on agency across disciplines. Our work explores increasingly agentic algorithmic systems in three parts. Rather, we use the term agency to highlight the increasingly evident fact that ML systems are not fully under human control. We emphasize that recognizing agency of algorithmic systems does not absolve or shift the human responsibility for algorithmic harms. We also discuss important harms which arise from increasing agency - notably, these include systemic and/or long-range impacts, often on marginalized stakeholders. Rather than providing a definition of agency as a binary property, we identify 4 key characteristics which, particularly in combination, tend to increase the agency of a given algorithmic system: underspecification, directness of impact, goal-directedness, and long-term planning. Our work focuses on the anticipation of harms from increasingly agentic systems. In response, the FATE community has emphasized the importance of anticipating harms. Despite these ongoing harms, new systems are being developed and deployed which threaten the perpetuation of the same harms and the creation of novel ones. Much work remains to be done to mitigate the serious harms of these systems, particularly those disproportionately affecting marginalized communities. Research in Fairness, Accountability, Transparency, and Ethics (FATE) has established many sources and forms of algorithmic harm, in domains as diverse as health care, finance, policing, and recommendations. In particular, compared with the same neural solvers under a standard training pipeline, ASP produces a remarkable decrease in terms of the optimality gap with 90.9% and 47.43% on generated instances and real-world instances for TSP, and a decrease of 19% and 45.57% for CVRP. Our results show that even with the same model size and weak training signal, ASP can help neural solvers explore and adapt to unseen distributions and varying scales, achieving superior performance. We have tested ASP on several challenging COPs, including the traveling salesman problem, the vehicle routing problem, and the prize collecting TSP, as well as the real-world instances from TSPLib and CVRPLib. ASP consists of two components: Distributional Exploration, which enhances the solver's ability to handle unknown distributions using Policy Space Response Oracles, and Persistent Scale Adaption, which improves scalability through curriculum learning. In this paper, we propose a new approach called ASP: Adaptive Staircase Policy Space Response Oracle to address these generalization issues and learn a universal neural solver.

However, existing learning-based solvers often struggle with generalization when faced with changes in problem distributions and scales.
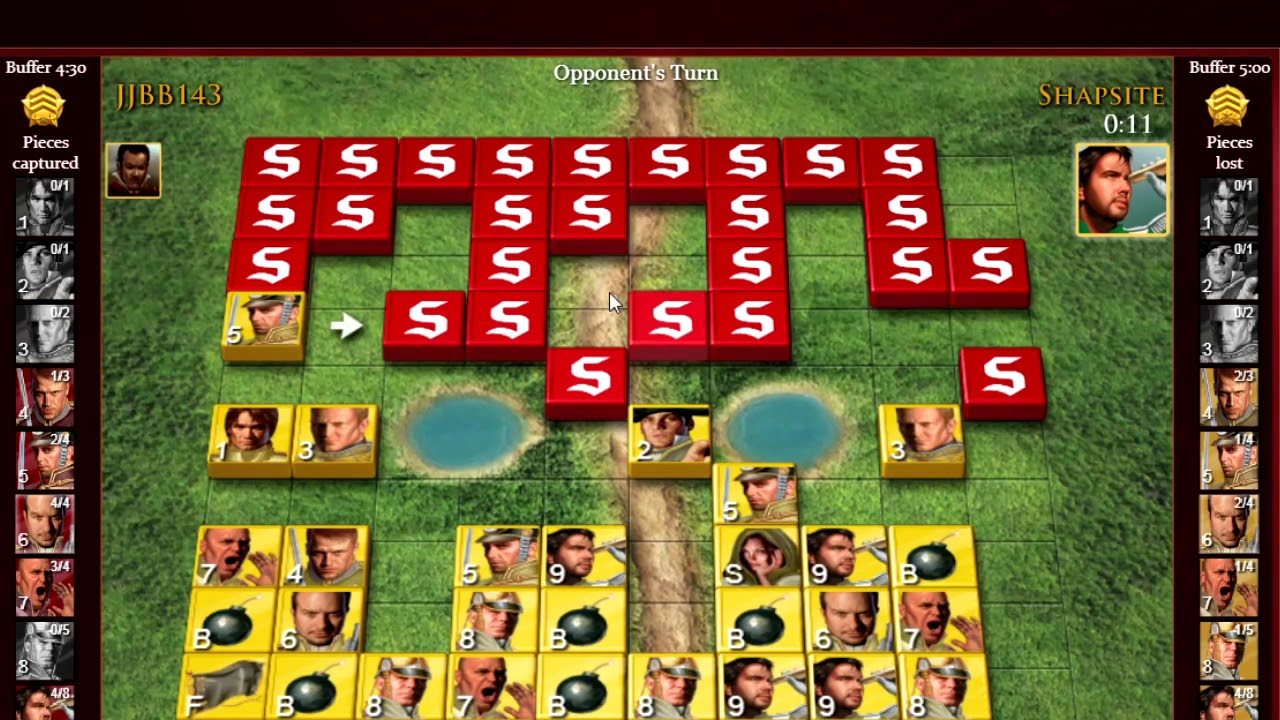
Applying machine learning to combinatorial optimization problems has the potential to improve both efficiency and accuracy.
